Beyond Prompt Engineering
The conversation around AI productivity has been dominated by prompting—the art of phrasing requests to get better outputs. While prompt engineering has its place, it's fundamentally a tactical approach to a strategic challenge. It treats each interaction as isolated and each output as an endpoint rather than part of an ongoing system.
Organizations that move beyond this limited view are discovering something powerful: the real leverage isn't in clever prompts. It's in deliberate structure. It's in building consistent frameworks that shape how information flows between human and machine intelligence—not just once, but over time.
This shift from prompting to structuring represents a new discipline for AI collaboration—one that transforms episodic interactions into coherent systems of meaning.
The Limitations of the Perfect Prompt
The allure of the perfect prompt is understandable. There's something magical about finding exactly the right words to unlock an AI's capabilities. Social media abounds with "prompt hacks" promising extraordinary results if you just phrase things precisely right.
But this approach has severe limitations:
- It's inherently fragile—minor variations in wording can yield dramatically different results
- It treats each interaction as disconnected from those before and after
- It creates dependency on individual expertise rather than organizational capability
- It focuses on immediate outputs rather than long-term learning
Organizations still caught in the prompt optimization trap often develop elaborate "prompt libraries"—collections of effective phrasings for specific tasks. But while these libraries can produce good immediate results, they don't build lasting capability. They don't create systems that learn and improve. They don't scale.
The alternative is structure—deliberate frameworks that transcend individual prompts to shape how humans and AI systems exchange meaning over time.
How Structure Shapes Interpretation
When you provide information to an AI system, the structure of that information profoundly influences how it's interpreted. This holds true whether you're conscious of the structure or not.
Consider two ways of presenting the same business challenge:
Approach 1:
"Our revenue is down this quarter. What should we do?"
Approach 2:
Business Situation:
- Q3 revenue down 12% vs. forecast
Primary Areas of Concern:
- New customer acquisition rate down 18%
- Average contract value stable
- Renewal rate improved 3%
Business Objectives:
- Return to forecast by Q1
- Maintain profitability during recovery
- Protect team morale through challenging period
Constraints:
- Marketing budget fixed until next fiscal year
- Product release cycle is 8 weeks
- Sales team at capacity
What approaches should we consider to address our revenue shortfall while respecting these constraints and objectives?
Both prompts address the same basic question. But the structured approach does something far more powerful than just requesting a better answer. It teaches the AI system how your organization thinks—what you value, how you frame problems, what constraints matter to you.
This structure doesn't just improve the immediate output (though it certainly does that). It establishes a pattern of interaction that can be consistently applied across teams, allowing the organization to build collective capability rather than relying on individual prompt-crafting skill.
Reusable Frameworks Over Ad Hoc Queries
The most advanced organizations are moving beyond prompt optimization to framework development—creating standardized structures for different types of business activities.
For example, a strategic planning framework might include sections for:
- Current situation assessment
- Key metrics and trends
- Strategic options under consideration
- Evaluation criteria
- Decision timeline and process
A product development framework might structure information around:
- User need being addressed
- Current solutions and limitations
- Proposed approach
- Success criteria
- Testing methodology
A customer communication framework could include:
- Customer profile and history
- Specific trigger for communication
- Desired outcome
- Key points to include
- Tone and voice guidelines
These frameworks aren't just better prompts. They're thinking templates that create consistency across the organization. When everyone structures information in similar ways, both humans and AI systems develop shared understanding more quickly.
Template-Driven Thinking for Consistency
Frameworks become truly powerful when they're implemented as reusable templates—standard formats that teams can apply to different situations.
These templates serve several critical functions:
- They reduce cognitive load by providing clear starting points
- They improve output quality by ensuring comprehensive context
- They create consistency that allows for meaningful comparison
- They establish trackable patterns that support continuous improvement
- They enable knowledge transfer across teams
For example, a "customer objection response" template might include standard sections for capturing the customer's concern, clarifying underlying needs, connecting to relevant product capabilities, addressing specific objections, and suggesting next steps.
When this template is used consistently, it doesn't just produce better individual responses. It creates a structured dataset that allows the organization to analyze patterns in customer concerns and response effectiveness over time.
The Essential Trio: Context, Constraints, Objectives
Across different frameworks and templates, three elements consistently emerge as essential for effective AI collaboration:
Context provides the foundation—the situational understanding that frames everything else. It includes relevant history, current state, and key relationships. Without sufficient context, AI systems default to general patterns rather than targeted responses.
Constraints define the boundaries within which solutions must operate. These might be resource limitations, timing requirements, or policy guidelines. Articulating constraints prevents AI systems from suggesting impractical approaches and focuses creativity where it can be most productive.
Objectives clarify what success looks like. They transform vague aspirations into specific targets, allowing both humans and AI to evaluate potential approaches against clear criteria. Well-defined objectives prevent the "right answer to the wrong question" problem that plagues many AI interactions.
Together, these elements create a problem space within which productive collaboration can occur. This trio should be present in virtually every significant AI interaction—explicitly articulated rather than assumed.
Miscommunication as Signal, Not Failure
When structuring AI interactions, we need to recognize that miscommunications aren't simply failures. They're valuable signals about gaps in our shared understanding.
In traditional approaches, an AI response that misses the mark is treated as a disappointment—something to be fixed through better prompting. In a structured approach, it becomes diagnostic information—revealing areas where frameworks need refinement or where assumptions aren't aligned.
This perspective transforms how we respond to suboptimal interactions:
- Instead of simply trying different wording, we analyze which structural elements were missing or misinterpreted
- Instead of treating each miscommunication as an isolated incident, we look for patterns across interactions
- Instead of focusing solely on improving immediate outputs, we use insights to refine our underlying frameworks
By treating miscommunication as signal rather than noise, we create learning systems that continuously improve not just individual interactions but our entire approach to collaboration.
Semantic Clarity: The Foundation of Long-Term Value
One of the most overlooked benefits of structured AI interaction is semantic clarity—the consistent use of terminology and concepts across an organization.
When teams develop shared vocabulary for discussing key ideas, several powerful things happen:
- Knowledge transfer becomes more efficient
- Misunderstandings decrease
- Pattern recognition improves
- Institutional memory strengthens
- AI systems learn more effectively
This clarity doesn't happen by accident. It requires deliberate attention to how concepts are named, defined, and related to each other. It means choosing specific terms for important ideas and using them consistently across teams and systems.
For example, rather than variously referring to "customers," "clients," "accounts," and "users" (creating confusion about whether these are distinct or overlapping groups), an organization might establish clear definitions and relationships between these terms, then use them precisely in all AI interactions.
This semantic hygiene might seem pedantic, but it creates enormous long-term value. It allows AI systems to recognize patterns more effectively, connect related information more accurately, and develop increasingly nuanced understanding of your specific business context.
Interfaces That Listen, Not Just Execute
A fundamental shift in structured collaboration is moving from interfaces that merely execute commands to interfaces that actively listen and learn.
Traditional prompt engineering treats AI as a command-response system—you issue carefully crafted instructions and receive outputs. Structured collaboration establishes a more sophisticated relationship where the AI system:
- Recognizes patterns in how you frame problems
- Builds understanding of your specific organizational context
- Adapts to your terminology and conceptual frameworks
- Learns from feedback what approaches resonate with your needs
- Develops increasing alignment with your objectives over time
This shift requires thinking beyond isolated interactions to ongoing relationships. It means designing not just for immediate utility but for progressive development of shared understanding.
In practice, this might involve:
- Establishing consistent structures for different types of interactions
- Creating explicit feedback mechanisms that capture more than binary success/failure
- Building institutional memory through structured documentation of effective approaches
- Developing shared language for key concepts and processes
- Creating spaces for reflection on what's working and what needs adjustment
The goal is an interface that doesn't just passively receive instructions but actively participates in the collaboration—listening, learning, and adapting over time.
Reducing Variance Through Structure
One of the most immediate benefits of structured AI collaboration is reduced variance in outputs. When information is presented consistently, results become more predictable—not in a constraining way, but in a reliability-enhancing way.
This consistency is particularly valuable in enterprise environments where:
- Multiple team members need comparable results
- Outputs inform important decisions
- Quality standards must be maintained at scale
- Results need to be repeatable over time
The traditional approach to reducing variance is reviewing and editing AI outputs after they're generated. But this is inherently inefficient—it treats symptoms rather than causes.
Structured collaboration addresses the root issue by providing clear, comprehensive context from the beginning. When AI systems receive consistently structured inputs, they produce more consistently valuable outputs, reducing the need for extensive post-production review.
Feedback Systems as Core Infrastructure
Effective AI collaboration isn't just about how information flows from humans to machines. It's equally about how feedback flows in the other direction.
Advanced organizations are building feedback mechanisms directly into their collaboration frameworks—systematically capturing what worked, what didn't, and why. This transforms isolated interactions into learning systems that improve over time.
Effective feedback structures:
- Establish clear evaluation criteria aligned with business objectives
- Create simple mechanisms for rating outputs (not just binary good/bad judgments)
- Capture specific improvement suggestions
- Track patterns across multiple interactions
- Feed insights back into framework refinement
For example, a team using AI for customer communications might rate each generated message on several dimensions: alignment with brand voice, factual accuracy, persuasiveness, and clarity. These ratings, along with specific comments, create a dataset that reveals patterns—which types of communications are consistently strong, which need improvement, and what factors predict success.
Over time, this structured feedback allows the organization to refine both its templates and its evaluation criteria, creating a continuous improvement loop.
Aligning HubSpot and AI Workflows Through Templates
For organizations using HubSpot, the connection between CRM structure and AI effectiveness creates powerful opportunities.
When HubSpot fields, properties, and workflows align with AI collaboration templates, information flows seamlessly between systems. Data captured in structured customer interactions automatically populates relevant HubSpot fields, and CRM data automatically enriches AI interactions.
This alignment requires deliberate design—mapping how information should flow between systems and ensuring semantic consistency across platforms. But the returns are substantial: reduced manual data entry, more comprehensive context for AI interactions, and richer customer data for analysis.
For example, a "sales call summary" template might include sections for customer concerns, product interests, next steps, and follow-up timeline. When this template aligns with HubSpot fields, information captured during the call flows automatically into the customer record, and future AI interactions are automatically enriched with this historical context.
This integration transforms both systems. HubSpot becomes more than a database—it becomes an evolving repository of institutional knowledge that continuously enhances AI interactions. And AI becomes more than a conversational interface—it becomes a structured way of capturing and applying customer insights.
Creating Repeatable Success Through Structured Dialogue
The ultimate goal of this structural approach is creating repeatable success—the ability to consistently achieve valuable outcomes from AI collaboration regardless of which team members are involved or which specific tasks are being addressed.
This repeatability comes from several reinforcing elements:
- Consistent information architecture across interactions
- Shared vocabulary for key concepts
- Standard templates for common activities
- Integrated feedback systems for continuous improvement
- Aligned workflows between AI and other business systems
Together, these elements create an environment where AI collaboration becomes a reliable capability rather than a hit-or-miss exercise in prompt crafting.
The shift from prompting to structuring doesn't mean abandoning creativity or flexibility. On the contrary, good structure creates space for meaningful exploration by establishing clear foundations. Just as musical structure (rhythm, harmony, key) enables rather than constrains artistic expression, information structure enables rather than constrains productive collaboration.
Building Your Structural Foundation
If you're ready to move beyond prompt optimization toward structured AI collaboration, here are key steps to consider:
- Audit your most common AI use cases: Identify the interactions that happen most frequently and have the greatest business impact.
- Develop standardized frameworks for these high-value activities, focusing on the context-constraints-objectives trio.
- Create reusable templates that implement these frameworks in practical formats your team can easily adopt.
- Establish semantic guidelines for key concepts, ensuring consistent terminology across teams and systems.
- Build feedback mechanisms that systematically capture what's working and what needs improvement.
- Align your AI structures with HubSpot, particularly your custom properties, workflows, and knowledge management approaches.
- Train your team not just in using these structures but in understanding why they matter.
This foundation won't be built overnight. But each step creates immediate value while contributing to longer-term capability. And unlike investments in specific AI tools or techniques (which may become obsolete), investments in structured collaboration create enduring advantage.
The Discipline of Structured Collaboration
Prompting is a skill. Structuring is a discipline.
Skills help individuals perform specific tasks more effectively. Disciplines transform how entire organizations operate.
As AI becomes increasingly central to business operations, the organizations that thrive won't be those with a few prompt engineering experts. They'll be those that develop structural disciplines—consistent approaches to how information flows between human and machine intelligence.
These disciplines won't just produce better immediate outputs. They'll create learning systems that continuously improve. They'll establish patterns that transfer knowledge more effectively. They'll build bridges between AI and other business systems.
In short, they'll transform AI from a useful tool into a fundamental business capability—one that's woven into the fabric of how the organization thinks and operates.
The prompt era is ending. The art of steering era has begun.
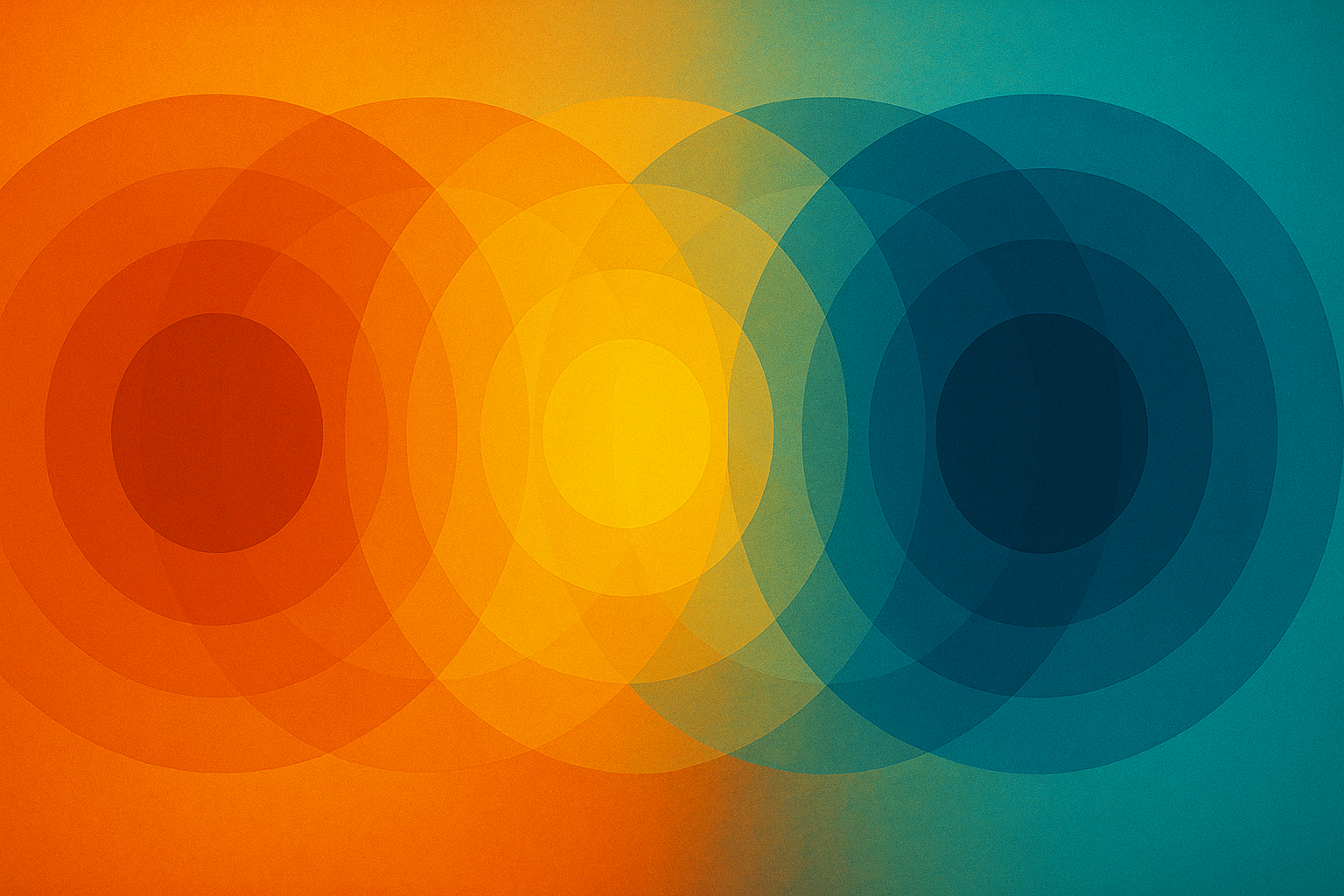
Beyond Prompt Engineering
The conversation around AI productivity has been dominated by prompting—the art of phrasing requests to get better outputs. While prompt engineering has its place, it's fundamentally a tactical approach to a strategic challenge. It treats each interaction as isolated and each output as an endpoint rather than part of an ongoing system.
Organizations that move beyond this limited view are discovering something powerful: the real leverage isn't in clever prompts. It's in deliberate structure. It's in building consistent frameworks that shape how information flows between human and machine intelligence—not just once, but over time.
This shift from prompting to structuring represents a new discipline for AI collaboration—one that transforms episodic interactions into coherent systems of meaning.
The Limitations of the Perfect Prompt
The allure of the perfect prompt is understandable. There's something magical about finding exactly the right words to unlock an AI's capabilities. Social media abounds with "prompt hacks" promising extraordinary results if you just phrase things precisely right.
But this approach has severe limitations:
- It's inherently fragile—minor variations in wording can yield dramatically different results
- It treats each interaction as disconnected from those before and after
- It creates dependency on individual expertise rather than organizational capability
- It focuses on immediate outputs rather than long-term learning
Organizations still caught in the prompt optimization trap often develop elaborate "prompt libraries"—collections of effective phrasings for specific tasks. But while these libraries can produce good immediate results, they don't build lasting capability. They don't create systems that learn and improve. They don't scale.
The alternative is structure—deliberate frameworks that transcend individual prompts to shape how humans and AI systems exchange meaning over time.
How Structure Shapes Interpretation
When you provide information to an AI system, the structure of that information profoundly influences how it's interpreted. This holds true whether you're conscious of the structure or not.
Consider two ways of presenting the same business challenge:
Approach 1:
"Our revenue is down this quarter. What should we do?"
Approach 2:
Business Situation:
- Q3 revenue down 12% vs. forecast
Primary Areas of Concern:
- New customer acquisition rate down 18%
- Average contract value stable
- Renewal rate improved 3%
Business Objectives:
- Return to forecast by Q1
- Maintain profitability during recovery
- Protect team morale through challenging period
Constraints:
- Marketing budget fixed until next fiscal year
- Product release cycle is 8 weeks
- Sales team at capacity
What approaches should we consider to address our revenue shortfall while respecting these constraints and objectives?
Both prompts address the same basic question. But the structured approach does something far more powerful than just requesting a better answer. It teaches the AI system how your organization thinks—what you value, how you frame problems, what constraints matter to you.
This structure doesn't just improve the immediate output (though it certainly does that). It establishes a pattern of interaction that can be consistently applied across teams, allowing the organization to build collective capability rather than relying on individual prompt-crafting skill.
Reusable Frameworks Over Ad Hoc Queries
The most advanced organizations are moving beyond prompt optimization to framework development—creating standardized structures for different types of business activities.
For example, a strategic planning framework might include sections for:
- Current situation assessment
- Key metrics and trends
- Strategic options under consideration
- Evaluation criteria
- Decision timeline and process
A product development framework might structure information around:
- User need being addressed
- Current solutions and limitations
- Proposed approach
- Success criteria
- Testing methodology
A customer communication framework could include:
- Customer profile and history
- Specific trigger for communication
- Desired outcome
- Key points to include
- Tone and voice guidelines
These frameworks aren't just better prompts. They're thinking templates that create consistency across the organization. When everyone structures information in similar ways, both humans and AI systems develop shared understanding more quickly.
Template-Driven Thinking for Consistency
Frameworks become truly powerful when they're implemented as reusable templates—standard formats that teams can apply to different situations.
These templates serve several critical functions:
- They reduce cognitive load by providing clear starting points
- They improve output quality by ensuring comprehensive context
- They create consistency that allows for meaningful comparison
- They establish trackable patterns that support continuous improvement
- They enable knowledge transfer across teams
For example, a "customer objection response" template might include standard sections for capturing the customer's concern, clarifying underlying needs, connecting to relevant product capabilities, addressing specific objections, and suggesting next steps.
When this template is used consistently, it doesn't just produce better individual responses. It creates a structured dataset that allows the organization to analyze patterns in customer concerns and response effectiveness over time.
The Essential Trio: Context, Constraints, Objectives
Across different frameworks and templates, three elements consistently emerge as essential for effective AI collaboration:
Context provides the foundation—the situational understanding that frames everything else. It includes relevant history, current state, and key relationships. Without sufficient context, AI systems default to general patterns rather than targeted responses.
Constraints define the boundaries within which solutions must operate. These might be resource limitations, timing requirements, or policy guidelines. Articulating constraints prevents AI systems from suggesting impractical approaches and focuses creativity where it can be most productive.
Objectives clarify what success looks like. They transform vague aspirations into specific targets, allowing both humans and AI to evaluate potential approaches against clear criteria. Well-defined objectives prevent the "right answer to the wrong question" problem that plagues many AI interactions.
Together, these elements create a problem space within which productive collaboration can occur. This trio should be present in virtually every significant AI interaction—explicitly articulated rather than assumed.
Miscommunication as Signal, Not Failure
When structuring AI interactions, we need to recognize that miscommunications aren't simply failures. They're valuable signals about gaps in our shared understanding.
In traditional approaches, an AI response that misses the mark is treated as a disappointment—something to be fixed through better prompting. In a structured approach, it becomes diagnostic information—revealing areas where frameworks need refinement or where assumptions aren't aligned.
This perspective transforms how we respond to suboptimal interactions:
- Instead of simply trying different wording, we analyze which structural elements were missing or misinterpreted
- Instead of treating each miscommunication as an isolated incident, we look for patterns across interactions
- Instead of focusing solely on improving immediate outputs, we use insights to refine our underlying frameworks
By treating miscommunication as signal rather than noise, we create learning systems that continuously improve not just individual interactions but our entire approach to collaboration.
Semantic Clarity: The Foundation of Long-Term Value
One of the most overlooked benefits of structured AI interaction is semantic clarity—the consistent use of terminology and concepts across an organization.
When teams develop shared vocabulary for discussing key ideas, several powerful things happen:
- Knowledge transfer becomes more efficient
- Misunderstandings decrease
- Pattern recognition improves
- Institutional memory strengthens
- AI systems learn more effectively
This clarity doesn't happen by accident. It requires deliberate attention to how concepts are named, defined, and related to each other. It means choosing specific terms for important ideas and using them consistently across teams and systems.
For example, rather than variously referring to "customers," "clients," "accounts," and "users" (creating confusion about whether these are distinct or overlapping groups), an organization might establish clear definitions and relationships between these terms, then use them precisely in all AI interactions.
This semantic hygiene might seem pedantic, but it creates enormous long-term value. It allows AI systems to recognize patterns more effectively, connect related information more accurately, and develop increasingly nuanced understanding of your specific business context.
Interfaces That Listen, Not Just Execute
A fundamental shift in structured collaboration is moving from interfaces that merely execute commands to interfaces that actively listen and learn.
Traditional prompt engineering treats AI as a command-response system—you issue carefully crafted instructions and receive outputs. Structured collaboration establishes a more sophisticated relationship where the AI system:
- Recognizes patterns in how you frame problems
- Builds understanding of your specific organizational context
- Adapts to your terminology and conceptual frameworks
- Learns from feedback what approaches resonate with your needs
- Develops increasing alignment with your objectives over time
This shift requires thinking beyond isolated interactions to ongoing relationships. It means designing not just for immediate utility but for progressive development of shared understanding.
In practice, this might involve:
- Establishing consistent structures for different types of interactions
- Creating explicit feedback mechanisms that capture more than binary success/failure
- Building institutional memory through structured documentation of effective approaches
- Developing shared language for key concepts and processes
- Creating spaces for reflection on what's working and what needs adjustment
The goal is an interface that doesn't just passively receive instructions but actively participates in the collaboration—listening, learning, and adapting over time.
Reducing Variance Through Structure
One of the most immediate benefits of structured AI collaboration is reduced variance in outputs. When information is presented consistently, results become more predictable—not in a constraining way, but in a reliability-enhancing way.
This consistency is particularly valuable in enterprise environments where:
- Multiple team members need comparable results
- Outputs inform important decisions
- Quality standards must be maintained at scale
- Results need to be repeatable over time
The traditional approach to reducing variance is reviewing and editing AI outputs after they're generated. But this is inherently inefficient—it treats symptoms rather than causes.
Structured collaboration addresses the root issue by providing clear, comprehensive context from the beginning. When AI systems receive consistently structured inputs, they produce more consistently valuable outputs, reducing the need for extensive post-production review.
Feedback Systems as Core Infrastructure
Effective AI collaboration isn't just about how information flows from humans to machines. It's equally about how feedback flows in the other direction.
Advanced organizations are building feedback mechanisms directly into their collaboration frameworks—systematically capturing what worked, what didn't, and why. This transforms isolated interactions into learning systems that improve over time.
Effective feedback structures:
- Establish clear evaluation criteria aligned with business objectives
- Create simple mechanisms for rating outputs (not just binary good/bad judgments)
- Capture specific improvement suggestions
- Track patterns across multiple interactions
- Feed insights back into framework refinement
For example, a team using AI for customer communications might rate each generated message on several dimensions: alignment with brand voice, factual accuracy, persuasiveness, and clarity. These ratings, along with specific comments, create a dataset that reveals patterns—which types of communications are consistently strong, which need improvement, and what factors predict success.
Over time, this structured feedback allows the organization to refine both its templates and its evaluation criteria, creating a continuous improvement loop.
Aligning HubSpot and AI Workflows Through Templates
For organizations using HubSpot, the connection between CRM structure and AI effectiveness creates powerful opportunities.
When HubSpot fields, properties, and workflows align with AI collaboration templates, information flows seamlessly between systems. Data captured in structured customer interactions automatically populates relevant HubSpot fields, and CRM data automatically enriches AI interactions.
This alignment requires deliberate design—mapping how information should flow between systems and ensuring semantic consistency across platforms. But the returns are substantial: reduced manual data entry, more comprehensive context for AI interactions, and richer customer data for analysis.
For example, a "sales call summary" template might include sections for customer concerns, product interests, next steps, and follow-up timeline. When this template aligns with HubSpot fields, information captured during the call flows automatically into the customer record, and future AI interactions are automatically enriched with this historical context.
This integration transforms both systems. HubSpot becomes more than a database—it becomes an evolving repository of institutional knowledge that continuously enhances AI interactions. And AI becomes more than a conversational interface—it becomes a structured way of capturing and applying customer insights.
Creating Repeatable Success Through Structured Dialogue
The ultimate goal of this structural approach is creating repeatable success—the ability to consistently achieve valuable outcomes from AI collaboration regardless of which team members are involved or which specific tasks are being addressed.
This repeatability comes from several reinforcing elements:
- Consistent information architecture across interactions
- Shared vocabulary for key concepts
- Standard templates for common activities
- Integrated feedback systems for continuous improvement
- Aligned workflows between AI and other business systems
Together, these elements create an environment where AI collaboration becomes a reliable capability rather than a hit-or-miss exercise in prompt crafting.
The shift from prompting to structuring doesn't mean abandoning creativity or flexibility. On the contrary, good structure creates space for meaningful exploration by establishing clear foundations. Just as musical structure (rhythm, harmony, key) enables rather than constrains artistic expression, information structure enables rather than constrains productive collaboration.
Building Your Structural Foundation
If you're ready to move beyond prompt optimization toward structured AI collaboration, here are key steps to consider:
- Audit your most common AI use cases: Identify the interactions that happen most frequently and have the greatest business impact.
- Develop standardized frameworks for these high-value activities, focusing on the context-constraints-objectives trio.
- Create reusable templates that implement these frameworks in practical formats your team can easily adopt.
- Establish semantic guidelines for key concepts, ensuring consistent terminology across teams and systems.
- Build feedback mechanisms that systematically capture what's working and what needs improvement.
- Align your AI structures with HubSpot, particularly your custom properties, workflows, and knowledge management approaches.
- Train your team not just in using these structures but in understanding why they matter.
This foundation won't be built overnight. But each step creates immediate value while contributing to longer-term capability. And unlike investments in specific AI tools or techniques (which may become obsolete), investments in structured collaboration create enduring advantage.
The Discipline of Structured Collaboration
Prompting is a skill. Structuring is a discipline.
Skills help individuals perform specific tasks more effectively. Disciplines transform how entire organizations operate.
As AI becomes increasingly central to business operations, the organizations that thrive won't be those with a few prompt engineering experts. They'll be those that develop structural disciplines—consistent approaches to how information flows between human and machine intelligence.
These disciplines won't just produce better immediate outputs. They'll create learning systems that continuously improve. They'll establish patterns that transfer knowledge more effectively. They'll build bridges between AI and other business systems.
In short, they'll transform AI from a useful tool into a fundamental business capability—one that's woven into the fabric of how the organization thinks and operates.
The prompt era is ending. The art of steering era has begun.