In our exploration of the Art of Steering framework, we've examined how a well-designed vehicle creates the foundation for effective movement and how environmental awareness enables responsive navigation. Now we turn to the third critical node: the Goal.
The goal represents your intended destination or desired outcome—the direction toward which all steering efforts are oriented. Without clear goals, even the most sophisticated vehicle operating in a well-understood environment will wander aimlessly, achieving little of lasting value.
In the age of AI, goal-setting takes on new dimensions. AI systems don't inherently understand your intentions or values—they require explicit direction to align their capabilities with your desired outcomes. At the same time, their analytical power can help refine goals, test their feasibility, and track progress with unprecedented precision.
Consider the sophistication of modern navigational systems as a metaphor for understanding effective goal-setting. From ancient star charts to GPS satellites, navigation represents humanity's enduring quest to establish and reach meaningful destinations. Modern navigation combines precise destination coordinates with flexible routing, constant position-fixing, and multiple reference frames to ensure reliable progress toward intended objectives.
Let's explore the four essential clusters that create effective goal orientation:
Targeting & Focus Tools: Defining What You're Aiming At
At the foundation of effective goal-setting is clarity about what you're targeting and how precisely you're focusing your efforts. This fundamental orientation determines whether your energy disperses across too many objectives or concentrates with appropriate intensity on priority outcomes.
Shotgun vs. Rifle
This concept addresses the spectrum of focus width—from broad, dispersed attention (shotgun) to narrow, concentrated focus (rifle). Neither approach is inherently superior; each serves different purposes in different contexts.
In AI collaboration, this distinction becomes critical for how you direct AI resources. Shotgun approaches might leverage AI for broad pattern detection across diverse data sets, while rifle approaches might deploy focused AI analysis on specific high-value problems.
Implementation Tip: Audit your current AI implementations to identify whether you're using a shotgun or rifle approach. Are you deploying AI broadly across many use cases with moderate impact, or concentrating on fewer high-value applications? For mature organisations, a portfolio approach with 70% rifle (focused applications) and 30% shotgun (broader exploration) often provides the best balance between immediate impact and future opportunity discovery.
Linear
Linear thinking addresses sequential relationships between goals—how earlier achievements enable later ones, creating dependencies and progression paths. Linear goal structures create clarity about prerequisites, staging, and developmental sequences.
AI can enhance linear goal structures by identifying optimal sequences, predicting completion timelines, and detecting potential bottlenecks in dependency chains. This analytical support creates more realistic linear planning than would be possible through intuition alone.
Implementation Tip: Use AI planning tools to create more sophisticated linear goal sequences in your CRM implementation. For example, when designing nurture paths or onboarding sequences, use AI analysis to identify the optimal ordering of content and touchpoints rather than relying on assumptions. Test different sequences with small samples to validate the AI-recommended linear paths before full deployment.
Intensity
Intensity represents the concentration of effort, attention, and resources applied to goal pursuit. High-intensity focus creates momentum and breakthrough progress but may be difficult to sustain; lower intensity approaches may enable longer-term consistent advancement.
AI systems can help optimize intensity by identifying when concentrated effort will create disproportionate returns versus when more distributed attention is appropriate. This dynamic intensity management creates more efficient resource allocation than fixed intensity levels.
Implementation Tip: Implement AI-driven "intensity signaling" in your marketing and sales operations. Use pattern analysis to identify when prospects are showing heightened interest or entering critical decision windows, then automatically increase engagement intensity during these periods. This dynamic approach creates more appropriate resource allocation than fixed contact schedules.
Progress
Progress measures advancement toward goals, creating feedback about whether efforts are producing desired movement. Well-designed progress metrics create motivation, direction, and accountability without becoming ends in themselves.
AI dramatically enhances progress tracking by enabling more sophisticated measurement models, real-time monitoring, and predictive indicators that anticipate future advancement. These capabilities create richer progress awareness than traditional lagging metrics alone.
Implementation Tip: Move beyond simple milestone tracking to AI-enhanced progress monitoring. For complex goals like customer success or digital transformation, develop composite progress indicators that combine multiple signals rather than relying on single metrics. Use AI to weight these factors appropriately and identify leading indicators that predict future progress before it becomes visible in outcome measures.
Momentum
Momentum represents the self-reinforcing quality of movement—how current progress creates energy and capability for continued advancement. Positive momentum makes further progress easier; negative momentum (stalling) creates increasing resistance to movement.
AI can help identify momentum patterns by analyzing not just absolute progress but acceleration or deceleration rates across key indicators. These insights enable more proactive intervention before negative momentum becomes entrenched.
Implementation Tip: Implement momentum monitoring in your sales pipeline management. Beyond tracking static conversion rates, use AI to analyze rate-of-change patterns that indicate building or diminishing momentum. Configure alerts when deal momentum shows early warning signs of stalling, enabling proactive intervention before opportunities become jeopardized.
Outcome Modeling & Evaluation: Anticipating and Measuring Results
Setting meaningful goals requires the ability to anticipate potential outcomes, model different scenarios, and establish appropriate evaluation mechanisms. This forward-looking perspective ensures that goals align with genuine value creation rather than merely driving activity.
Trajectory
Trajectory represents the projected path from current position to desired outcome. Understanding trajectory involves not just the destination but the likely route, including potential obstacles, acceleration points, and required course corrections.
AI enhances trajectory modeling through more sophisticated predictive analytics, accounting for more variables and identifying non-obvious factors that might influence your path. These capabilities create more realistic projections than simplified linear extrapolation.
Implementation Tip: Use AI-powered trajectory modeling when setting growth targets in your CRM. Rather than simple linear projections, develop models that account for seasonality, market dynamics, competitive factors, and internal capacity constraints. These multi-factor projections create more achievable targets than aspirational numbers disconnected from likely pathways.
Digital Twin/Modeling
This concept involves creating virtual representations of systems or processes to simulate potential outcomes before real-world implementation. Digital twins enable low-risk experimentation, scenario testing, and optimization without the costs or consequences of physical trial-and-error.
AI dramatically expands modeling capabilities through more sophisticated simulation engines, the ability to incorporate more variables, and computational power that enables testing many more scenarios than would be practical manually.
Implementation Tip: Before implementing major CRM workflow changes, create AI-powered simulation models that test different configurations with historical data. This "digital twin" approach allows you to predict how changes will affect key metrics like lead conversion or customer retention before making changes in your production environment, substantially reducing implementation risk.
Proof of Concept
Proof of concept involves small-scale testing to validate assumptions before full investment. These limited implementations provide real-world feedback about whether a proposed approach is likely to deliver expected outcomes when scaled.
AI can enhance proof of concept testing through more sophisticated analysis of test results, identification of non-obvious patterns in limited data sets, and projection of how findings might translate to larger implementations.
Implementation Tip: Design AI-enhanced A/B testing that goes beyond simple conversion comparison to deeper pattern analysis. Use AI to identify which customer segments respond most positively to new approaches, what contextual factors influence results, and how initial responses predict longer-term outcomes. These richer insights create more reliable scaling decisions than aggregate performance metrics alone.
Derivative
The derivative concept focuses on rate of change rather than absolute values—how quickly metrics are improving or declining rather than their current level. This perspective highlights acceleration, deceleration, and inflection points that might be missed when focusing only on current position.
AI systems excel at derivative analysis through their ability to detect subtle changes in rate patterns, identify when trends are beginning to shift, and distinguish between temporary fluctuations and meaningful direction changes.
Implementation Tip: Implement "rate of change" dashboards for your key CRM metrics. Beyond showing current values, use AI analysis to highlight acceleration or deceleration patterns and flag early warning signs of trend shifts. Configure alerts when critical metrics show significant changes in direction, enabling proactive response before negative trends become established.
Signals
Signals are indicators that provide information about progress, position, or potential issues. Effective goal-setting includes identifying what signals will be monitored, how they'll be interpreted, and what thresholds will trigger response.
AI dramatically enhances signal processing through the ability to monitor more indicators simultaneously, detect subtle patterns across multiple signals, and distinguish meaningful signals from noise with greater precision than human perception alone.
Implementation Tip: Use AI-powered signal aggregation to create more sophisticated early warning systems for customer health monitoring. Rather than relying on a few obvious metrics like login frequency or support tickets, incorporate dozens of subtle signals that collectively predict retention risk. Use AI weighting to create composite indicators that provide reliable alerts before problems become obvious in traditional metrics.
Strategic Goal Framing: Connecting Outcomes to Value
Beyond specific targets, effective goal-setting requires strategic framing that connects immediate objectives to lasting value creation. This broader perspective ensures that achievements matter beyond simply hitting metrics, creating genuine advancement rather than isolated successes.
Return on Investment (ROI)
ROI frames goals in terms of value created relative to resources invested. This perspective ensures that achievements aren't evaluated in isolation but in context of what was required to attain them.
AI enhances ROI analysis through more comprehensive accounting of both investments and returns, including factors that might be overlooked in traditional calculations. These capabilities create more accurate value assessment than simplified financial metrics alone.
Implementation Tip: Implement AI-enhanced ROI modeling for your marketing activities that goes beyond simple attribution models. Use multi-touch, time-decay approaches that account for both direct and nurturing contributions across the full customer journey. This sophisticated approach creates more accurate investment decisions than models that oversimplify the complex pathways to conversion.
North Star
A North Star metric serves as the primary orientation point that aligns all other goals and activities. Like celestial navigation, this guiding reference creates consistency of direction despite changing conditions or immediate pressures.
AI can support North Star alignment by tracking how various activities and metrics contribute to the primary objective, identifying potential conflicts between immediate targets and the guiding metric, and suggesting adjustments to maintain overall direction.
Implementation Tip: Once you've established your North Star metric, use AI analysis to evaluate how effectively your current CRM configuration supports it. Do your workflows, automations, and reporting directly advance this primary objective, or have they drifted toward other purposes? This alignment analysis often reveals opportunities to simplify processes that don't directly contribute to your guiding metric.
Cumulative and Churn
This concept addresses how achievements accumulate or diminish over time—whether progress is truly additive or subject to erosion through churn, attrition, or decay. Understanding these dynamics prevents the illusion of advancement when gains in one area are offset by losses elsewhere.
AI enhances understanding of cumulative effects and churn through more sophisticated cohort analysis, detection of subtle retention patterns, and projection of long-term net effects beyond immediate gains or losses.
Implementation Tip: Implement AI-powered cohort analysis that tracks not just immediate conversion metrics but long-term retention and value patterns. For example, examine how different acquisition channels or onboarding approaches affect 12-month customer value, not just initial conversion rates. These longitudinal insights often reveal that what appears most effective in the short term may not create the greatest cumulative value.
Move the Needle
This concept focuses on meaningful impact versus incremental change—whether efforts create substantial advancement or merely minor improvements. This perspective prevents the dispersion of resources across too many initiatives with insufficient individual impact.
AI helps identify "needle-moving" opportunities through impact analysis that predicts which potential initiatives are likely to create significant advancement versus marginal gains. These projections enable more strategic resource allocation than distributing effort evenly across all possibilities.
Implementation Tip: Use AI predictive modeling to assess which potential CRM enhancements would create the most significant impact on key business outcomes. Rather than implementing a long list of minor improvements, focus resources on the few changes that modeling suggests will "move the needle" on critical metrics. This concentrated approach often delivers greater overall advancement than distributing effort across many incremental optimizations.
Diminishing Returns
Diminishing returns recognizes that continued investment in any area eventually produces decreasing benefit per unit of additional resource. Understanding these thresholds prevents overinvestment in activities that have passed their optimal efficiency point.
AI excels at identifying diminishing returns patterns through analysis of historical performance curves, detection of inflection points where efficiency declines, and prediction of optimal investment levels before returns begin to diminish.
Implementation Tip: Apply AI analysis to identify diminishing returns thresholds in your marketing activities. For example, determine at what point additional email frequency begins to reduce overall engagement rather than increase it, or when additional fields in forms start to significantly reduce completion rates. These insights enable optimization at the peak efficiency point rather than wasteful overinvestment.
Goal Alignment with Stakeholders: Creating Shared Direction
Even the most perfectly crafted goals create little value if they don't resonate with stakeholders whose support and engagement are necessary for success. This final cluster addresses how goals connect with the people and groups whose participation enables achievement.
Retention & Loyalty
This concept addresses how goals relate to keeping and deepening existing relationships rather than just acquiring new ones. This perspective prevents the common pattern of focusing on growth at the expense of maintaining and developing current connections.
AI enhances retention and loyalty strategies through more sophisticated prediction of churn risk, identification of engagement patterns that indicate relationship strength, and personalized approaches to relationship development at scale.
Implementation Tip: Implement AI-powered "relationship health scoring" that goes beyond basic usage metrics to identify the emotional and commitment dimensions of customer relationships. Use natural language processing to analyze support interactions, linguistic patterns in communications, and engagement consistency. These deeper indicators often reveal relationship strengths or vulnerabilities that transaction metrics miss.
Referrals
Referrals represent stakeholder advocacy that extends your reach through trusted relationships. Goal alignment with referral psychology ensures that achievements naturally generate advocacy rather than requiring separate promotion efforts.
AI can enhance referral strategies by identifying the optimal moments for referral requests, predicting which customers are most likely to become advocates, and personalizing advocacy programs based on individual motivations.
Implementation Tip: Rather than uniform referral programs, use AI pattern analysis to develop targeted advocacy approaches based on customer behavior profiles. Some customers respond best to recognition incentives, others to financial rewards, and still others to exclusive access. Use AI to match these motivation patterns with appropriate advocacy programs rather than one-size-fits-all approaches.
Survey
Surveys provide structured feedback about stakeholder perceptions, preferences, and experiences. Well-designed survey strategies create systematic understanding of how goals and their pursuit are perceived by critical audiences.
AI dramatically enhances survey approaches through more sophisticated analysis of response patterns, identification of non-obvious correlations between different feedback elements, and the ability to extract insights from unstructured comments beyond quantitative ratings.
Implementation Tip: Use AI-powered text analysis for open-ended survey responses rather than focusing primarily on numerical ratings. Natural language processing can identify sentiment patterns, recurring themes, and emotional intensity that quantitative measures miss. These qualitative insights often reveal the "why" behind score changes that would otherwise remain mysterious.
Spread
Spread addresses how widely goals and their associated behaviors diffuse through stakeholder communities. Understanding spread dynamics ensures that achievements extend beyond initial adoption to create broader impact.
AI enhances spread analysis through social network modeling, adoption pattern recognition, and identification of the factors that accelerate or inhibit diffusion. These capabilities create more effective spread strategies than intuitive approaches alone.
Implementation Tip: When introducing new CRM features or processes, use AI adoption analysis to identify the specific factors that predict successful uptake versus resistance. Rather than assuming all users respond to the same motivators, identify the distinct adoption patterns for different team segments. These insights enable targeted change management rather than uniform approaches that often fail with certain user groups.
Radar
Radar represents systematic scanning for signals that might affect goal relevance, feasibility, or approach. This forward-looking awareness ensures that goals adapt to emerging conditions rather than becoming outdated or irrelevant.
AI significantly enhances radar capabilities through continuous monitoring across more information sources, pattern recognition that identifies subtle early signals, and predictive analytics that connect current indicators to future developments.
Implementation Tip: Implement an AI-powered "goal relevance radar" that continuously scans for developments that might affect your strategic objectives. Configure monitoring for competitive moves, regulatory changes, technology developments, and market shifts that could impact your direction. Set up regular review sessions to evaluate these signals and assess whether goal adjustments are warranted.
Integration: Creating a Coherent Goal System
While we've explored these clusters individually, their true power emerges through integration. Effective goal-setting isn't just about establishing individual targets but creating a coherent system where objectives work together to drive meaningful advancement.
Consider how these clusters interact:
- Targeting and focus establish the foundation—determining what you're aiming at and how precisely you're directing effort.
- Outcome modeling and evaluation create realistic expectations—ensuring goals are achievable and their achievement can be meaningfully assessed.
- Strategic framing connects immediate objectives to lasting value—preventing the pursuit of metrics that don't create genuine advancement.
- Stakeholder alignment ensures necessary support and engagement—recognizing that goals require shared direction to translate into shared achievement.
Together, these elements create what we might call a goal ecosystem—a set of interconnected objectives that collectively guide the organization toward meaningful outcomes while adapting to changing conditions and stakeholder needs.
Setting Goals in the Age of AI
As you develop your organization's approach to goal-setting in AI-enhanced environments, consider these key principles:
- Balance precision with adaptability. Goals should be specific enough to provide clear direction but flexible enough to accommodate changing conditions. Use AI to model potential scenarios and develop contingency approaches rather than rigid targets that become irrelevant when circumstances change.
- Create multi-dimensional success metrics. Move beyond single-variable targets to more sophisticated goal models that track multiple dimensions of success. AI can help manage the complexity of these richer models while still providing clear guidance for day-to-day decisions.
- Connect activities to outcomes. Ensure that measurable targets directly link to genuine value creation rather than becoming ends in themselves. Use AI to model the relationship between activity metrics and outcome measures to prevent optimization for metrics that don't create meaningful results.
- Build in continuous relevance testing. Establish regular review processes that evaluate whether goals remain appropriate as conditions evolve. Use AI to monitor signals that might suggest the need for goal adjustment rather than persisting with outdated objectives.
- Design for both human motivation and machine direction. Goals in AI-enhanced systems need to simultaneously inspire people and guide algorithms. Develop objective frameworks that provide both the emotional resonance humans need and the precision machines require.
By thoughtfully developing your goal-setting capabilities—from targeting to stakeholder alignment—you create the essential directional clarity for effective steering in the age of AI. Even the most sophisticated vehicle navigating a well-understood environment will make little meaningful progress without clear destination coordinates.
Want to assess your current goal-setting approach and identify opportunities for enhancement? Contact me to discuss how these principles could be applied in your specific business context.
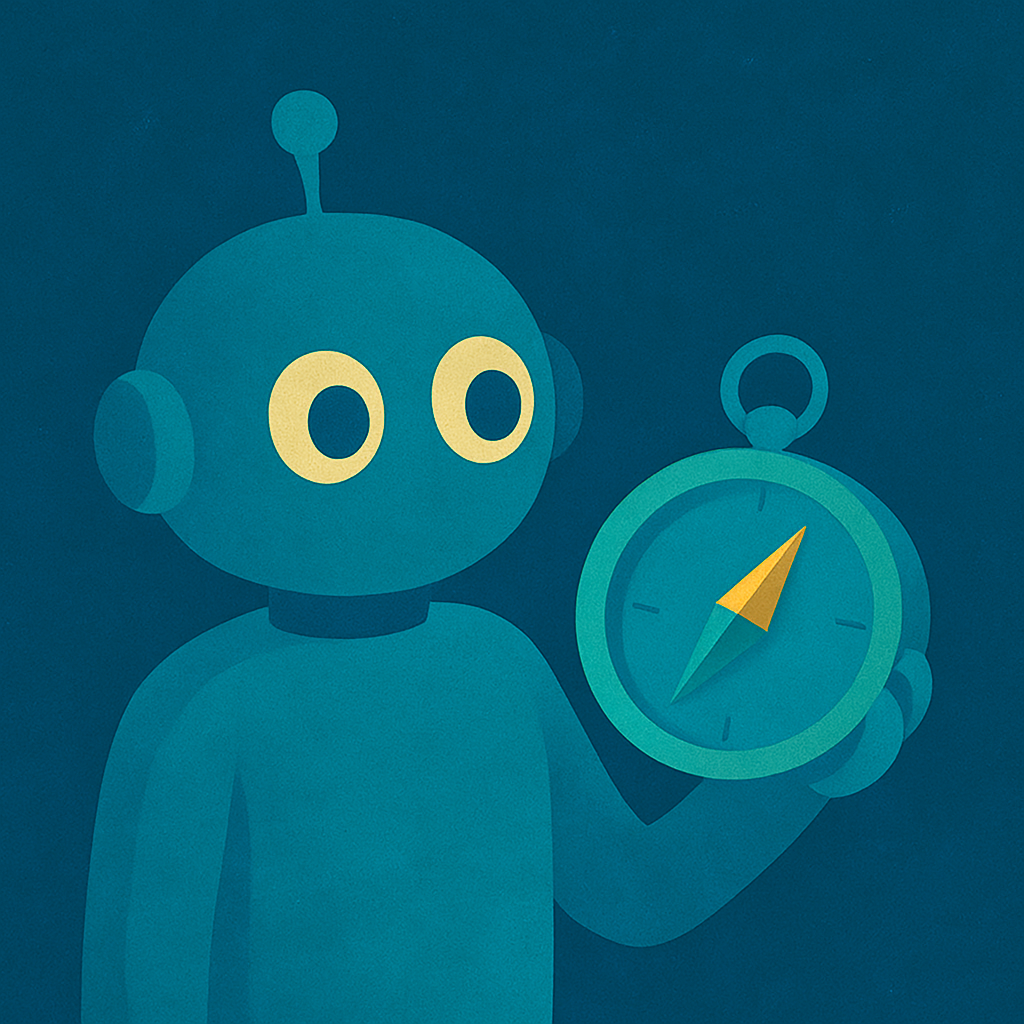
In our exploration of the Art of Steering framework, we've examined how a well-designed vehicle creates the foundation for effective movement and how environmental awareness enables responsive navigation. Now we turn to the third critical node: the Goal.
The goal represents your intended destination or desired outcome—the direction toward which all steering efforts are oriented. Without clear goals, even the most sophisticated vehicle operating in a well-understood environment will wander aimlessly, achieving little of lasting value.
In the age of AI, goal-setting takes on new dimensions. AI systems don't inherently understand your intentions or values—they require explicit direction to align their capabilities with your desired outcomes. At the same time, their analytical power can help refine goals, test their feasibility, and track progress with unprecedented precision.
Consider the sophistication of modern navigational systems as a metaphor for understanding effective goal-setting. From ancient star charts to GPS satellites, navigation represents humanity's enduring quest to establish and reach meaningful destinations. Modern navigation combines precise destination coordinates with flexible routing, constant position-fixing, and multiple reference frames to ensure reliable progress toward intended objectives.
Let's explore the four essential clusters that create effective goal orientation:
Targeting & Focus Tools: Defining What You're Aiming At
At the foundation of effective goal-setting is clarity about what you're targeting and how precisely you're focusing your efforts. This fundamental orientation determines whether your energy disperses across too many objectives or concentrates with appropriate intensity on priority outcomes.
Shotgun vs. Rifle
This concept addresses the spectrum of focus width—from broad, dispersed attention (shotgun) to narrow, concentrated focus (rifle). Neither approach is inherently superior; each serves different purposes in different contexts.
In AI collaboration, this distinction becomes critical for how you direct AI resources. Shotgun approaches might leverage AI for broad pattern detection across diverse data sets, while rifle approaches might deploy focused AI analysis on specific high-value problems.
Implementation Tip: Audit your current AI implementations to identify whether you're using a shotgun or rifle approach. Are you deploying AI broadly across many use cases with moderate impact, or concentrating on fewer high-value applications? For mature organisations, a portfolio approach with 70% rifle (focused applications) and 30% shotgun (broader exploration) often provides the best balance between immediate impact and future opportunity discovery.
Linear
Linear thinking addresses sequential relationships between goals—how earlier achievements enable later ones, creating dependencies and progression paths. Linear goal structures create clarity about prerequisites, staging, and developmental sequences.
AI can enhance linear goal structures by identifying optimal sequences, predicting completion timelines, and detecting potential bottlenecks in dependency chains. This analytical support creates more realistic linear planning than would be possible through intuition alone.
Implementation Tip: Use AI planning tools to create more sophisticated linear goal sequences in your CRM implementation. For example, when designing nurture paths or onboarding sequences, use AI analysis to identify the optimal ordering of content and touchpoints rather than relying on assumptions. Test different sequences with small samples to validate the AI-recommended linear paths before full deployment.
Intensity
Intensity represents the concentration of effort, attention, and resources applied to goal pursuit. High-intensity focus creates momentum and breakthrough progress but may be difficult to sustain; lower intensity approaches may enable longer-term consistent advancement.
AI systems can help optimize intensity by identifying when concentrated effort will create disproportionate returns versus when more distributed attention is appropriate. This dynamic intensity management creates more efficient resource allocation than fixed intensity levels.
Implementation Tip: Implement AI-driven "intensity signaling" in your marketing and sales operations. Use pattern analysis to identify when prospects are showing heightened interest or entering critical decision windows, then automatically increase engagement intensity during these periods. This dynamic approach creates more appropriate resource allocation than fixed contact schedules.
Progress
Progress measures advancement toward goals, creating feedback about whether efforts are producing desired movement. Well-designed progress metrics create motivation, direction, and accountability without becoming ends in themselves.
AI dramatically enhances progress tracking by enabling more sophisticated measurement models, real-time monitoring, and predictive indicators that anticipate future advancement. These capabilities create richer progress awareness than traditional lagging metrics alone.
Implementation Tip: Move beyond simple milestone tracking to AI-enhanced progress monitoring. For complex goals like customer success or digital transformation, develop composite progress indicators that combine multiple signals rather than relying on single metrics. Use AI to weight these factors appropriately and identify leading indicators that predict future progress before it becomes visible in outcome measures.
Momentum
Momentum represents the self-reinforcing quality of movement—how current progress creates energy and capability for continued advancement. Positive momentum makes further progress easier; negative momentum (stalling) creates increasing resistance to movement.
AI can help identify momentum patterns by analyzing not just absolute progress but acceleration or deceleration rates across key indicators. These insights enable more proactive intervention before negative momentum becomes entrenched.
Implementation Tip: Implement momentum monitoring in your sales pipeline management. Beyond tracking static conversion rates, use AI to analyze rate-of-change patterns that indicate building or diminishing momentum. Configure alerts when deal momentum shows early warning signs of stalling, enabling proactive intervention before opportunities become jeopardized.
Outcome Modeling & Evaluation: Anticipating and Measuring Results
Setting meaningful goals requires the ability to anticipate potential outcomes, model different scenarios, and establish appropriate evaluation mechanisms. This forward-looking perspective ensures that goals align with genuine value creation rather than merely driving activity.
Trajectory
Trajectory represents the projected path from current position to desired outcome. Understanding trajectory involves not just the destination but the likely route, including potential obstacles, acceleration points, and required course corrections.
AI enhances trajectory modeling through more sophisticated predictive analytics, accounting for more variables and identifying non-obvious factors that might influence your path. These capabilities create more realistic projections than simplified linear extrapolation.
Implementation Tip: Use AI-powered trajectory modeling when setting growth targets in your CRM. Rather than simple linear projections, develop models that account for seasonality, market dynamics, competitive factors, and internal capacity constraints. These multi-factor projections create more achievable targets than aspirational numbers disconnected from likely pathways.
Digital Twin/Modeling
This concept involves creating virtual representations of systems or processes to simulate potential outcomes before real-world implementation. Digital twins enable low-risk experimentation, scenario testing, and optimization without the costs or consequences of physical trial-and-error.
AI dramatically expands modeling capabilities through more sophisticated simulation engines, the ability to incorporate more variables, and computational power that enables testing many more scenarios than would be practical manually.
Implementation Tip: Before implementing major CRM workflow changes, create AI-powered simulation models that test different configurations with historical data. This "digital twin" approach allows you to predict how changes will affect key metrics like lead conversion or customer retention before making changes in your production environment, substantially reducing implementation risk.
Proof of Concept
Proof of concept involves small-scale testing to validate assumptions before full investment. These limited implementations provide real-world feedback about whether a proposed approach is likely to deliver expected outcomes when scaled.
AI can enhance proof of concept testing through more sophisticated analysis of test results, identification of non-obvious patterns in limited data sets, and projection of how findings might translate to larger implementations.
Implementation Tip: Design AI-enhanced A/B testing that goes beyond simple conversion comparison to deeper pattern analysis. Use AI to identify which customer segments respond most positively to new approaches, what contextual factors influence results, and how initial responses predict longer-term outcomes. These richer insights create more reliable scaling decisions than aggregate performance metrics alone.
Derivative
The derivative concept focuses on rate of change rather than absolute values—how quickly metrics are improving or declining rather than their current level. This perspective highlights acceleration, deceleration, and inflection points that might be missed when focusing only on current position.
AI systems excel at derivative analysis through their ability to detect subtle changes in rate patterns, identify when trends are beginning to shift, and distinguish between temporary fluctuations and meaningful direction changes.
Implementation Tip: Implement "rate of change" dashboards for your key CRM metrics. Beyond showing current values, use AI analysis to highlight acceleration or deceleration patterns and flag early warning signs of trend shifts. Configure alerts when critical metrics show significant changes in direction, enabling proactive response before negative trends become established.
Signals
Signals are indicators that provide information about progress, position, or potential issues. Effective goal-setting includes identifying what signals will be monitored, how they'll be interpreted, and what thresholds will trigger response.
AI dramatically enhances signal processing through the ability to monitor more indicators simultaneously, detect subtle patterns across multiple signals, and distinguish meaningful signals from noise with greater precision than human perception alone.
Implementation Tip: Use AI-powered signal aggregation to create more sophisticated early warning systems for customer health monitoring. Rather than relying on a few obvious metrics like login frequency or support tickets, incorporate dozens of subtle signals that collectively predict retention risk. Use AI weighting to create composite indicators that provide reliable alerts before problems become obvious in traditional metrics.
Strategic Goal Framing: Connecting Outcomes to Value
Beyond specific targets, effective goal-setting requires strategic framing that connects immediate objectives to lasting value creation. This broader perspective ensures that achievements matter beyond simply hitting metrics, creating genuine advancement rather than isolated successes.
Return on Investment (ROI)
ROI frames goals in terms of value created relative to resources invested. This perspective ensures that achievements aren't evaluated in isolation but in context of what was required to attain them.
AI enhances ROI analysis through more comprehensive accounting of both investments and returns, including factors that might be overlooked in traditional calculations. These capabilities create more accurate value assessment than simplified financial metrics alone.
Implementation Tip: Implement AI-enhanced ROI modeling for your marketing activities that goes beyond simple attribution models. Use multi-touch, time-decay approaches that account for both direct and nurturing contributions across the full customer journey. This sophisticated approach creates more accurate investment decisions than models that oversimplify the complex pathways to conversion.
North Star
A North Star metric serves as the primary orientation point that aligns all other goals and activities. Like celestial navigation, this guiding reference creates consistency of direction despite changing conditions or immediate pressures.
AI can support North Star alignment by tracking how various activities and metrics contribute to the primary objective, identifying potential conflicts between immediate targets and the guiding metric, and suggesting adjustments to maintain overall direction.
Implementation Tip: Once you've established your North Star metric, use AI analysis to evaluate how effectively your current CRM configuration supports it. Do your workflows, automations, and reporting directly advance this primary objective, or have they drifted toward other purposes? This alignment analysis often reveals opportunities to simplify processes that don't directly contribute to your guiding metric.
Cumulative and Churn
This concept addresses how achievements accumulate or diminish over time—whether progress is truly additive or subject to erosion through churn, attrition, or decay. Understanding these dynamics prevents the illusion of advancement when gains in one area are offset by losses elsewhere.
AI enhances understanding of cumulative effects and churn through more sophisticated cohort analysis, detection of subtle retention patterns, and projection of long-term net effects beyond immediate gains or losses.
Implementation Tip: Implement AI-powered cohort analysis that tracks not just immediate conversion metrics but long-term retention and value patterns. For example, examine how different acquisition channels or onboarding approaches affect 12-month customer value, not just initial conversion rates. These longitudinal insights often reveal that what appears most effective in the short term may not create the greatest cumulative value.
Move the Needle
This concept focuses on meaningful impact versus incremental change—whether efforts create substantial advancement or merely minor improvements. This perspective prevents the dispersion of resources across too many initiatives with insufficient individual impact.
AI helps identify "needle-moving" opportunities through impact analysis that predicts which potential initiatives are likely to create significant advancement versus marginal gains. These projections enable more strategic resource allocation than distributing effort evenly across all possibilities.
Implementation Tip: Use AI predictive modeling to assess which potential CRM enhancements would create the most significant impact on key business outcomes. Rather than implementing a long list of minor improvements, focus resources on the few changes that modeling suggests will "move the needle" on critical metrics. This concentrated approach often delivers greater overall advancement than distributing effort across many incremental optimizations.
Diminishing Returns
Diminishing returns recognizes that continued investment in any area eventually produces decreasing benefit per unit of additional resource. Understanding these thresholds prevents overinvestment in activities that have passed their optimal efficiency point.
AI excels at identifying diminishing returns patterns through analysis of historical performance curves, detection of inflection points where efficiency declines, and prediction of optimal investment levels before returns begin to diminish.
Implementation Tip: Apply AI analysis to identify diminishing returns thresholds in your marketing activities. For example, determine at what point additional email frequency begins to reduce overall engagement rather than increase it, or when additional fields in forms start to significantly reduce completion rates. These insights enable optimization at the peak efficiency point rather than wasteful overinvestment.
Goal Alignment with Stakeholders: Creating Shared Direction
Even the most perfectly crafted goals create little value if they don't resonate with stakeholders whose support and engagement are necessary for success. This final cluster addresses how goals connect with the people and groups whose participation enables achievement.
Retention & Loyalty
This concept addresses how goals relate to keeping and deepening existing relationships rather than just acquiring new ones. This perspective prevents the common pattern of focusing on growth at the expense of maintaining and developing current connections.
AI enhances retention and loyalty strategies through more sophisticated prediction of churn risk, identification of engagement patterns that indicate relationship strength, and personalized approaches to relationship development at scale.
Implementation Tip: Implement AI-powered "relationship health scoring" that goes beyond basic usage metrics to identify the emotional and commitment dimensions of customer relationships. Use natural language processing to analyze support interactions, linguistic patterns in communications, and engagement consistency. These deeper indicators often reveal relationship strengths or vulnerabilities that transaction metrics miss.
Referrals
Referrals represent stakeholder advocacy that extends your reach through trusted relationships. Goal alignment with referral psychology ensures that achievements naturally generate advocacy rather than requiring separate promotion efforts.
AI can enhance referral strategies by identifying the optimal moments for referral requests, predicting which customers are most likely to become advocates, and personalizing advocacy programs based on individual motivations.
Implementation Tip: Rather than uniform referral programs, use AI pattern analysis to develop targeted advocacy approaches based on customer behavior profiles. Some customers respond best to recognition incentives, others to financial rewards, and still others to exclusive access. Use AI to match these motivation patterns with appropriate advocacy programs rather than one-size-fits-all approaches.
Survey
Surveys provide structured feedback about stakeholder perceptions, preferences, and experiences. Well-designed survey strategies create systematic understanding of how goals and their pursuit are perceived by critical audiences.
AI dramatically enhances survey approaches through more sophisticated analysis of response patterns, identification of non-obvious correlations between different feedback elements, and the ability to extract insights from unstructured comments beyond quantitative ratings.
Implementation Tip: Use AI-powered text analysis for open-ended survey responses rather than focusing primarily on numerical ratings. Natural language processing can identify sentiment patterns, recurring themes, and emotional intensity that quantitative measures miss. These qualitative insights often reveal the "why" behind score changes that would otherwise remain mysterious.
Spread
Spread addresses how widely goals and their associated behaviors diffuse through stakeholder communities. Understanding spread dynamics ensures that achievements extend beyond initial adoption to create broader impact.
AI enhances spread analysis through social network modeling, adoption pattern recognition, and identification of the factors that accelerate or inhibit diffusion. These capabilities create more effective spread strategies than intuitive approaches alone.
Implementation Tip: When introducing new CRM features or processes, use AI adoption analysis to identify the specific factors that predict successful uptake versus resistance. Rather than assuming all users respond to the same motivators, identify the distinct adoption patterns for different team segments. These insights enable targeted change management rather than uniform approaches that often fail with certain user groups.
Radar
Radar represents systematic scanning for signals that might affect goal relevance, feasibility, or approach. This forward-looking awareness ensures that goals adapt to emerging conditions rather than becoming outdated or irrelevant.
AI significantly enhances radar capabilities through continuous monitoring across more information sources, pattern recognition that identifies subtle early signals, and predictive analytics that connect current indicators to future developments.
Implementation Tip: Implement an AI-powered "goal relevance radar" that continuously scans for developments that might affect your strategic objectives. Configure monitoring for competitive moves, regulatory changes, technology developments, and market shifts that could impact your direction. Set up regular review sessions to evaluate these signals and assess whether goal adjustments are warranted.
Integration: Creating a Coherent Goal System
While we've explored these clusters individually, their true power emerges through integration. Effective goal-setting isn't just about establishing individual targets but creating a coherent system where objectives work together to drive meaningful advancement.
Consider how these clusters interact:
- Targeting and focus establish the foundation—determining what you're aiming at and how precisely you're directing effort.
- Outcome modeling and evaluation create realistic expectations—ensuring goals are achievable and their achievement can be meaningfully assessed.
- Strategic framing connects immediate objectives to lasting value—preventing the pursuit of metrics that don't create genuine advancement.
- Stakeholder alignment ensures necessary support and engagement—recognizing that goals require shared direction to translate into shared achievement.
Together, these elements create what we might call a goal ecosystem—a set of interconnected objectives that collectively guide the organization toward meaningful outcomes while adapting to changing conditions and stakeholder needs.
Setting Goals in the Age of AI
As you develop your organization's approach to goal-setting in AI-enhanced environments, consider these key principles:
- Balance precision with adaptability. Goals should be specific enough to provide clear direction but flexible enough to accommodate changing conditions. Use AI to model potential scenarios and develop contingency approaches rather than rigid targets that become irrelevant when circumstances change.
- Create multi-dimensional success metrics. Move beyond single-variable targets to more sophisticated goal models that track multiple dimensions of success. AI can help manage the complexity of these richer models while still providing clear guidance for day-to-day decisions.
- Connect activities to outcomes. Ensure that measurable targets directly link to genuine value creation rather than becoming ends in themselves. Use AI to model the relationship between activity metrics and outcome measures to prevent optimization for metrics that don't create meaningful results.
- Build in continuous relevance testing. Establish regular review processes that evaluate whether goals remain appropriate as conditions evolve. Use AI to monitor signals that might suggest the need for goal adjustment rather than persisting with outdated objectives.
- Design for both human motivation and machine direction. Goals in AI-enhanced systems need to simultaneously inspire people and guide algorithms. Develop objective frameworks that provide both the emotional resonance humans need and the precision machines require.
By thoughtfully developing your goal-setting capabilities—from targeting to stakeholder alignment—you create the essential directional clarity for effective steering in the age of AI. Even the most sophisticated vehicle navigating a well-understood environment will make little meaningful progress without clear destination coordinates.
Want to assess your current goal-setting approach and identify opportunities for enhancement? Contact me to discuss how these principles could be applied in your specific business context.